Microbiomes exhibit fluid compositions and behaviours, presenting challenges to accurately predict their dynamics over time. However, in an open system characterised by relatively controlled environmental constraints, our research group together with collaborators has now demonstrated the ability to successfully forecast the future composition and dynamics of the resident microbial community.
Biological wastewater treatment plants based on the activated sludge process play pivotal roles in civil infrastructure, constituting a vital component for treating wastewater while also harbouring the potential to recover valuable resources. At the core of a typical biological wastewater treatment plant lies a microbial community that serves as the “engine”, catalysing the necessary biochemical reactions for the treatment process. Under specific conditions, the process selects for microorganisms with the ability to accumulate lipids, resulting in the formation of buoyant foam that floats to the top of treatment tanks, posing challenges to normal plant operations. However, this foam also represents a valuable resource due to its high lipid content, which can be harnessed as a substrate for biofuel production. Therefore, the prediction of foam dynamics represents a crucial aim for the effective and sustainable management of wastewater treatment plant operations.
Multiple factors influence the composition of microbial communities in biological wastewater treatment plants, including variations in the chemical and biological composition of the influent (dependent on the time of day or day of the week), temperature-driven seasonality, and rainfall. Ensuring that a plant operates within the specified operational ranges of its key parameters is essential, thereby minimising the environmental variability to which the microbial community is exposed. This aspect, coupled with the ease of sampling and the moderate species richness, positions biological wastewater treatment plants as an exemplary model system for conducting basic studies in microbial ecology and for developing analytical frameworks to predict microbial community dynamics.
The study of the lipid-rich foam communities in biological wastewater treatment plants has allowed our research group together with many international collaborators to develop system ecology methodologies aimed at unravelling fundamental aspects of such communities. On the methodology front, we have developed the necessary wet-lab and dry-lab methods for integrated meta-omic analyses comprising systematic metagenomic, metatranscriptomic, metaproteomic and metabolomic measurements. Using this toolbox, we discovered that the success of the dominant microbial population, i.e. Candidatus Microthrix parvicella, is linked to its ability to fine-tune its gene expression over time depending on resource, an attribute which even manifests itself as fine-scale phenotypic heterogeneity at the single-cell level. We have further identified keystone genes and linked keystone organisms which have a disproportionate effect on the functioning of these communities. More recently, time-series sampling (Figure 1) and integrated multi-omics have allowed us to unravel how these microbial communities respond to disturbances. The same analyses have revealed how mobile genetic elements (MGEs) shape antimicrobial resistance within the microbial community, and how the tracking of MGEs and corresponding CRISPR loci allow the construction of models with quite good predictive power over the community dynamics.
By building on this earlier modelling work, in our most recent study, the foam community data from a 14-month time-series served as the training set, while a purposeful selection of 21 additional foam samples from the same plant, collected over the subsequent five years, was used to evaluate the predictability of the microbial community dynamics. Employing integrated meta-omics enabled the quantification of the most informative macromolecules in the community, i.e. DNA, RNA, and proteins. A combination of mathematical and statistical methodologies was then applied to discern temporal patterns in the data, elucidating them through seasonal components and environmental variables. By extrapolating the identified patterns over the five years following the training data sampling period and by incorporating environmental variables, we successfully predicted the composition of the test samples.
Our study unveiled that nearly all temporal dynamics of the microbial community, as expressed through the levels of DNA, RNA, and proteins alongside environmental variables over time, could be summarised by 17 fundamental signals. This suggests that the events within the foam’s populations are driven by a relatively small pool of factors. Although these drivers are primarily attributed to seasonal variations, our study underscores the role of the immediate environmental conditions within the plant. Moreover, we extrapolated the identified signals, amalgamating them to predict DNA and RNA levels in future samples. A comparison of average trend levels between predictions and test samples yielded highly accurate trend predictions (Figure 2). However, the predictive performance diminished over the years, indicating a multi-annual trend that is challenging to capture from the training set and is likely influenced by the continuous input of new microbial populations.
The analytical framework developed in our study is transferable to other microbial communities, facilitating the identification of fundamental temporal patterns that might not be readily apparent. Furthermore, the accurate prediction of community dynamics over time, contingent on environmental factors, is deemed instrumental in effectively operating biological wastewater treatment plants and other biotechnologies relying on mixed microbial processes in the future. Given that the wastewater treatment plant was maintained within operational parameters to constrain environmental effects, not all communities in natural habitats may exhibit the same predictability – but this needs to be investigated. Additionally, the diminishing prediction ability over years underscores the necessity to update the model continuously with more data for accurate predictions.
The logical next step involves assessing whether additional data can enhance predictions for subsequent years and determining the generalisability of these findings to other plants. In essence, the development of sustainable biological wastewater treatment plants, focused on fully recycling waste material and rooted in microbial communities, hinges on our capacity to predict microbial dynamics over time. As demonstrated in our study, this capability is achievable through the application of models built on integrated, high-resolution meta-omics data.
Our most recent study marks a significant milestone in our research dedicated to lipid-accumulating organismal assemblies within biological wastewater treatment plants. These communities have proven to be an exemplary model system for developing and implementing molecular systems ecology approaches for microbiomes. This research trajectory commenced in 2010 through an ATTRACT programme grant from the Luxembourg National Research Fund (FNR), enabling our group to establish methodologies for integrated multi-omics in conjunction with a long-term sampling scheme. With additional support from two subsequent FNR CORE grants, our recent study heavily relies on the unique microbiome time-series we have cultivated, spanning over a decade, emphasizing the importance of prolonged time-series in microbial ecology. Our latest study addresses one of our work’s two ultimate objectives—precisely predicting the behaviour of microbiomes. The rational control of microbial communities now stands as our next crucial challenge.
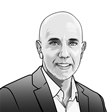
No comments yet